For years, marketers have used the analysis technique of media mix modeling (MMM) — also referred to as marketing mix modeling — to measure and compare the effectiveness of various marketing channels to help inform long-term planning and improve decision-making.
In this guide, we look at how MMM works and some of its key elements. We also assess its advantages and shortcomings, and explain how using call tracking technology to get full attribution for every media channel can deliver real value to your MMM and help increase your marketing return on investment (ROI).
What Is Media Mix Modeling (MMM)?
There is actually little difference between media mix modeling and marketing mix modeling; the former is really just a more modern label for the latter.
MMM is a method of analysing sales and marketing data that has been around for more than a half-century. In a modern marketing context, media mix modeling can be particularly useful to marketers because it offers an approach to measuring the performance of digital channels and offline performance.
MMM can provide high-level, holistic insight into marketing activities and performance by analysing the historical contributions of each media channel to overall sales. This creates a picture of the effectiveness of each channel and its impact on ROI that marketers can use predictively to make better decisions when planning future campaigns.
Media mix modeling could prove very useful to your business in the current economy in the same way that marketers have found the method helpful in trying to make sense of the COVID-19 pandemic’s impact on marketing performance. Honing your analysis of marketing spend and ROI before, during, and after a pre-recessionary economy can equip you with insights and tools to better prepare for and successfully navigate future downturns.
How Media Mix Modeling Works
MMM involves performing a statistical analysis using multiple linear regression to highlight the relationship between sales or conversions and ad spend. It takes historical aggregated data, typically two to three years’ worth, from marketing and non-marketing sources to identify precisely what causes sales.
Consider MMM the “50,000-foot view” of marketing effectiveness. Unlike most other marketing metrics, media mix modeling doesn’t utilise user-level data such as ad impressions or clicks. In short, it doesn’t measure the customer journey.
MMM instead provides a more holistic picture of the connection between marketing and sales performance, which can help inform strategic long-term planning. Showing which channels are working and which ones aren’t allows marketers to refine their strategies and deliver better outcomes from campaigns.
Consider this example: Say your automotive OEM (original equipment manufacturer) had $10 million in sales in the week of its 0% APR financing event. During that week, the marketing plan called for paid search ads, some regional TV ads in your top four markets of Seattle, San Francisco, New York, and Chicago, and social media outreach through Instagram posts and YouTube videos. You got a ton of test drives booked through your website, as well as over the phone.
By using digital media optimisation tools and MMM, you can determine how much of your total sales in that week were attributable to each marketing channel. The percentage of sales not attributable to any of those channels is your baseline, and it tells you what level of sales you might expect without doing any ads at all. You also have to account for your special financing event to make sure your overall analysis is accurate and not skewed to a major sales success.
For the MMM process, you can use data gathered from marketing channels and actions such as:
- Print and online display ads
- Paid search
- Direct mail
- Radio and TV ads
- Native content
- Social media
- Public relations and earned media
- Promotions
Additional information you might want to include in MMM could be seasonal factors such as holidays or established events (like the special financing offer in the example above). Weather conditions that might affect shopping patterns, and economic data such as unemployment rates, consumer confidence numbers, fuel prices, and inflation might also factor into your modeling process. Also, you could incorporate competitor data such as promotions and pricing, new product releases and advertising, and insights from actual customers gleaned from conversational analytics.
5 Key Elements Within Media Mix Modeling
These five elements should be incorporated into any MMM analysis:
1. Base and Incremental Sales
Media mix modeling can measure sales volume on both a base and incremental basis. Incremental sales are those in which marketing plays a role. Base sales are influenced by non-marketing-related factors such as seasonality, pricing, and branding.
2. Media and Ads
Incorporating data into MMM from media and ad buys can result in valuable comparisons of ad platforms and the impact of your ads. For example, you can compare the relative performance of ads on competing platforms such as Google and Facebook to discern if your target audience is more responsive on one versus the other.
3. Pricing
One of the biggest conundrums that marketers face is knowing when to adjust pricing, since any change up or down could critically impact sales, positively or negatively. MMM is useful here because it can help you pinpoint the impacts of price change strategies, allowing you to better understand the relationship between price changes at key moments for your brand.
4. Distribution
Here’s one that’s particularly important coming out of the global supply chain crisis fueled by the COVID-19 pandemic. We’ve all learned by now, if we didn’t know it before, that supply chain issues can have a dramatic impact on sales. Using distribution data gathered over the last two years in media mix modeling could be incredibly valuable for companies’ forward planning.
5. New Products
If you roll out a new product, you assume (and hope!) that it will have a positive impact on your company’s sales volume — but MMM can tell you exactly how much impact. This knowledge can inform the type and timing of future product launches.
Calculating the MMM Ratio
Media mix modeling tools include Python, a programming language useful for building media mix models. MMM employs multiple linear regression using dependent (sales) and independent (distribution, pricing, media spend) variables to come up with a linear or nonlinear equation that can be used to assign ratios to each marketing channel.
For more detail on using media mix modeling data science to calculate marketing’s impact on sales, check out the MMM primer provided in this post.
The Top 3 Advantages of Media Mix Modeling
Media Mix Modeling goes beyond simply measuring marketing performance. It acts as a strategic lens, offering a high-level overview across all marketing channels, revealing the intricate relationship between marketing efforts and sales performance. This comprehensive analysis unlocks valuable insights that empowers businesses to:
Advantage 1: Optimise Marketing Spend and Reduce Waste
Imagine a marketing budget spread thin across various channels, with limited understanding of which ones truly drive sales. Here's where media mix modeling shines. By analysing historical data, it identifies the most effective channels and quantifies their impact on sales. This allows businesses to:
- Target high-performing channels: Allocate resources strategically, focusing on channels demonstrably driving sales, and potentially reducing spending on less effective ones.
- Identify areas for improvement: Uncover channels with underperformance, prompting a deeper dive into their strategies and potential for optimisation.
- Navigate economic downturns: During recessions, when budgets tighten, MMM becomes even more crucial. It helps eliminate wasteful spending, ensuring marketing dollars are directed towards channels that deliver the most significant sales impact.
Advantage 2: Craft Winning Marketing Campaigns
MMM goes beyond basic attribution models, revealing the synergistic effects of different channels. Analysing how channels interact with each other provides a deeper understanding of the customer journey. Businesses can leverage these insights to:
- Develop data-driven campaign strategies: Design campaigns that combine the most impactful channels, creating a cohesive and effective marketing experience for customers.
- Test and refine cross-channel initiatives: MMM allows for evaluating the effectiveness of combined marketing efforts, leading to the development of more successful multi-channel campaigns.
- Foster long-term brand building: By understanding how brand awareness campaigns on TV or radio interact with targeted online ads, companies can optimise the overall brand experience.
Advantage 3: Inform Long-Term Marketing Planning
Marketing decisions shouldn't be made in a vacuum. Media mix modeling helps businesses look beyond immediate results by enabling:
- Sales forecasting: By analysing historical data and the impact of marketing efforts, MMM provides valuable insights for predicting future sales trends.
- Strategic budget allocation: Armed with long-term forecasts, businesses can plan future marketing budgets with greater confidence, ensuring resources are optimised to achieve growth goals.
- Scenario planning and risk mitigation: Media mix modeling allows businesses to model various marketing scenarios, predicting the potential impact of budget changes or new marketing initiatives. This facilitates proactive risk management and strategic planning for long-term success.
4 Media Mix Modeling Limitations
Media Mix Modeling offers valuable insights for marketers, but it's important to understand its limitations before diving in. Here are some key shortcomings to consider:
Limitation 1: Lack of Granularity
MMM analyses overall sales data, providing a high-level view of marketing performance. However, it doesn't capture the individual customer journey. Unlike other attribution models that leverage personal-level data, media mix modeling can't pinpoint the exact touchpoints that influenced a specific purchase decision. This makes it difficult to assess the effectiveness of highly targeted campaigns or those focused on specific audience segments.
Limitation 2: Historical Data Dependence
Media mix modeling relies heavily on historical data to build its models. If your business is relatively new or lacks at least two to three years of consistent marketing and sales data, MMM might not be the most effective tool. Without a substantial historical record, the model may struggle to identify accurate cause-and-effect relationships between marketing activities and sales performance.
Limitation 3: Limited Agility
Building and refining a media mix model can be a time-consuming process. This makes it less suitable for situations requiring ongoing, real-time optimisation. While MMM can provide valuable insights into long-term trends, it may not be ideal for teams needing to quickly adjust and optimise campaigns based on immediate performance data.
Limitation 4: Adaptability
The marketing landscape is constantly evolving, with new channels emerging and consumer behavior shifting. MMM models can struggle to adapt to these rapid changes. If significant disruptions occur, such as a new competitor entering the market or a major social media platform altering its algorithm, the model's accuracy might diminish, requiring updates and recalibration.
6 Steps to Implement Media Mix Modeling
So, you’ve decided to dive into media mix modeling because guessing your marketing ROI based on gut feelings and crossed fingers isn’t exactly a solid strategy. Here’s how we suggest you get started:
1. Gather Your Data (Yes, All of It)
Before you can start modeling, you need a mountain of data—historical marketing spend, conversions, website traffic, economic trends, competitor activity, and basically anything else that might influence consumer behavior. Think of it as detective work, but instead of solving a crime, you’re figuring out why people actually buy your product.
Fair warning: this step can feel overwhelming and tedious—because, well, it is. Tracking down disparate data sources, cleaning messy spreadsheets, and making sure everything aligns isn’t exactly a thrill ride. But here’s the thing: once you get past the initial grunt work, you’ll have all the insights to make every marketing decision smarter and more strategic. So yes, it’s a heavy lift, but one that’s absolutely worth the effort.
2. Define Your Goals. Otherwise, What’s the Point?
Are you trying to prove the return on your direct mailers? Do you want to see if paid search ads drive more leads than TV commercials? Do you want to measure how your social media ads translate to sales? Clearly define what success looks like—whether it’s sales, leads, or brand awareness—so your model doesn’t turn into an expensive guessing game.
3. Choose Your Model Because One Size Doesn’t Fit All
Not all models are created equal. Linear regression is the classic go-to, but if you’re feeling fancy, you might venture into Bayesian models or machine learning approaches. Just remember: a more complex model doesn’t always mean better results—sometimes, simplicity wins.
4. Account for External Factors
Your marketing doesn’t operate in a vacuum. Economic shifts, competitor moves, seasonal trends, and even the occasional PR disaster can mess with your results. Make sure your model considers these external forces, or you’ll be left wondering why your sales tanked after a viral PR scandal.
5. Test, Adjust, Repeat. The First Try Is Never Perfect.
MMM isn’t a "set it and forget it" situation. Once you’ve built your model, test it against real-world results, tweak it as needed, and then test again. It’s an ongoing cycle, much like your caffeine intake.
6. Translate Your Data into Recommendations
Great, you’ve got insights! But if you throw a bunch of numbers at leadership without context, prepare for glazed-over stares. Translate your findings into actionable recommendations that non-data folks can actually understand. Bonus points if you can prove that, yes, your department deserves more budget.
Improve Media Mix Modeling With Invoca
Media mix modeling is most successful when marketing receives full attribution for the campaigns it develops that drive sales. It’s easy to miss the full picture on attribution, though, especially when a high proportion of sales may be happening offline via phone calls to your contact centre.

Marketing attribution solutions can help fill the gap in attribution models by using call tracking to identify conversions made over the phone. With Invoca’s call tracking software, for example, you can easily develop a complete picture of your true marketing performance and the conversions your media channels are driving by including outcomes from both calls and digital clicks. This makes attribution modeling easier, and it can help you defend your marketing spend, too.
Additional Reading
Want to learn more about how Invoca can help you track the phone leads driven by your media campaigns, and decrease cost per acquisition? Check out these resources:
- How to Execute High-Impact Marketing Campaigns (with Examples!)
- How to Measure the Success of Marketing Campaigns
- The Dos and Don’ts of Marketing During a Recession
Request your personal demo to see how Invoca can help you improve your media mix modeling.
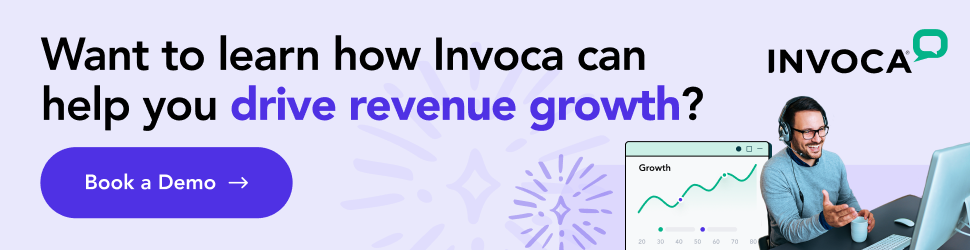